The Smart Energy Research Group from the Department of Electrical Engineering at Tsinghua University published an 18-page research paper titled “Data-driven Security and Stability Rule in High Renewable Penetrated Power System Operation” in the special issue “The Evolution of Smart Grids” of IEEE’s flagship journal Proceedings of the IEEE. This issue was led by Chongqing Kang, Chair of the Department of Electrical Engineering and Director of the Energy Internet Research Institute of Tsinghua University, and co-organized with Professor Timothy C. Green from Imperial College London and Professor Daniel S. Kirschen from The University of Washington. The paper’s first author and corresponding author was Ning Zhang, an associate professor in the Department of Electrical Engineering and the Head of the Energy Internet Coordination & Planning Research Office at the Energy Strategy and Operation Research Center of the Energy Internet Research Institute.
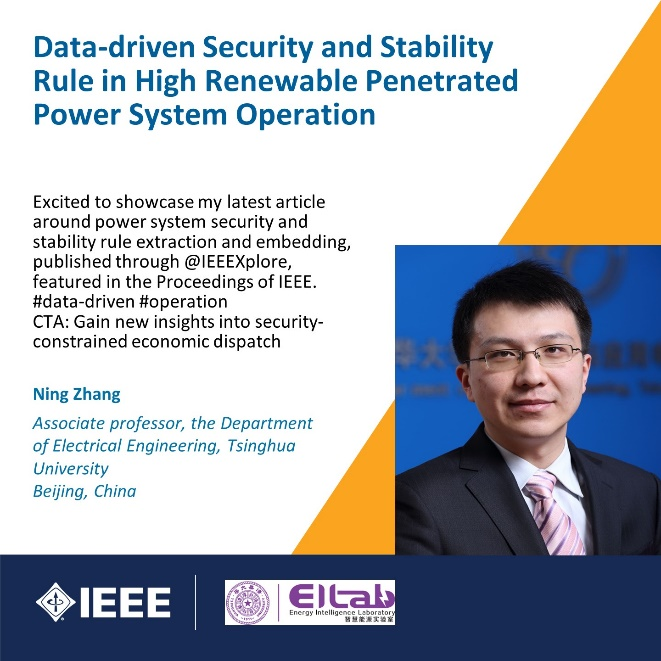
Publicity page on the Proceedings of the IEEE
One of the main characteristics of new power systems is the high penetration of renewable energy sources, which creates two challenges for new power systems: On one hand, the diverse generation units introduce large randomness and uncertainty to the power grid dispatching operation, and the typical operation modes of power systems increase, making the traditional method unable to ensure the long-term and short-term power balance simultaneously; On the other hand, the highly penetrated renewable energy sources are connected to power systems through power electronic devices, which complicates the security and stability mechanism of power systems and poses huge challenges to the secure and stable operation of power systems.
In this context, this paper proposes a set of data-driven methods for extracting and embedding security and stability rules in power system operations with high penetration of renewable energy sources. The methods generate massive operation scenarios and highly secure and stable data sets through simulation, learn the security and stability rules of power systems based on interpretable data-driven models, depict analytical security and stability boundaries of safe power systems, and then transform high-accuracy data-driven models into rules that can be embedded to optimize power system operation through convex linearization or the introduction of 0-1 variables. Security and stability constraints are considered in the operation optimization to ensure the secure and stable operation of systems.
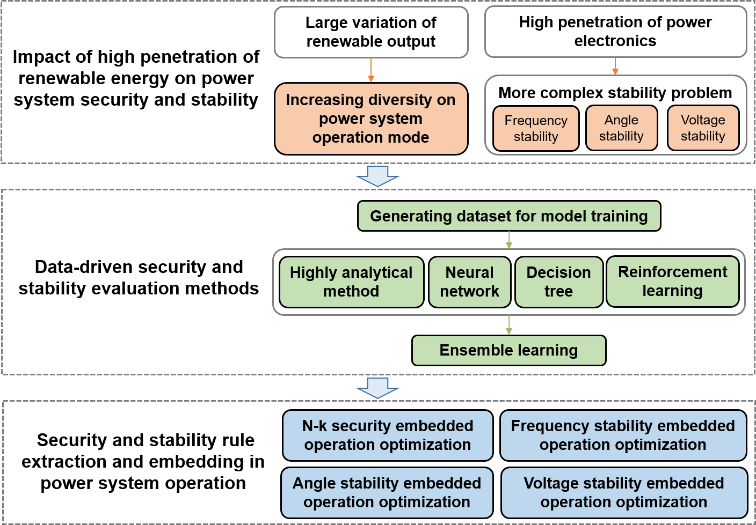
The research framework of the paper
This paper first examines the impact of high penetration of renewable energies on the security and stability of power systems, highlighting the effects on frequency stability, voltage stability, power angle stability and other issues. Then, it presents the data-driven methods for security and stability assessment of power systems, with an emphasis on various machine learning methods that can be used to identify the security and stability boundaries of power systems. Finally, it proposes a method for converting machine learning models into security and stability constraints that can be embedded for optimal operation, targeting different machine learning models. The proposed method has been verified through actual power system simulation that it can enhance the operational security and stability of power systems.
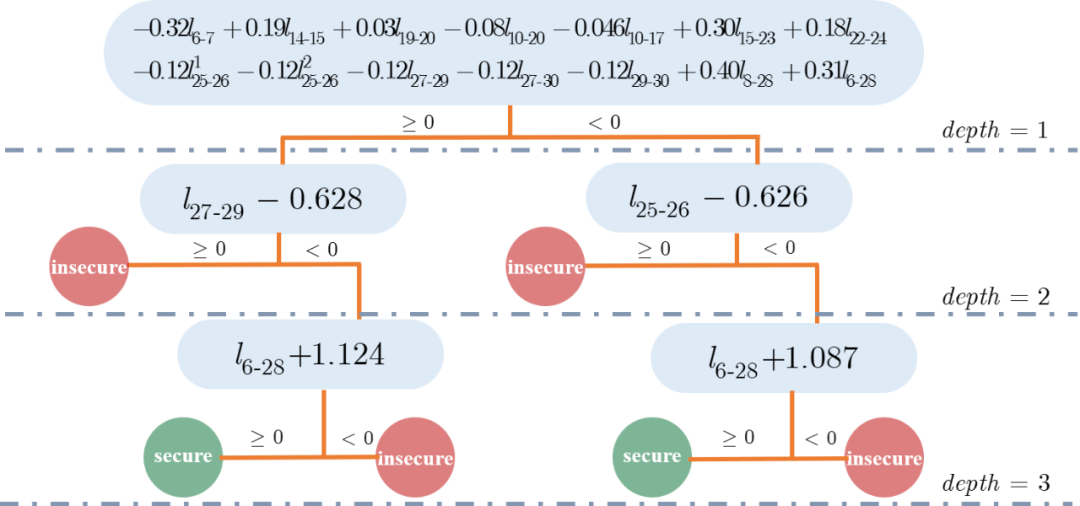
Schematic of security and stability rule extraction results for power systems
The conclusion of the paper summarizes two advantages of the proposed approach. First, the data-driven method is highly adaptable and can address various security and stability issues in different new power systems. Second, the embedded security and stability rules do not affect the data model of the conventional power system optimized operation, and the data-driven method can be smoothly integrated into the existing method framework. The paper also anticipates four directions for the future development of the proposed method: exploring more interpretable machine learning models, combining data-driven models with model-driven methods, ensuring the security and stability of systems in optimization, and enhancing the generalization capability of data-driven rules in optimal operation.
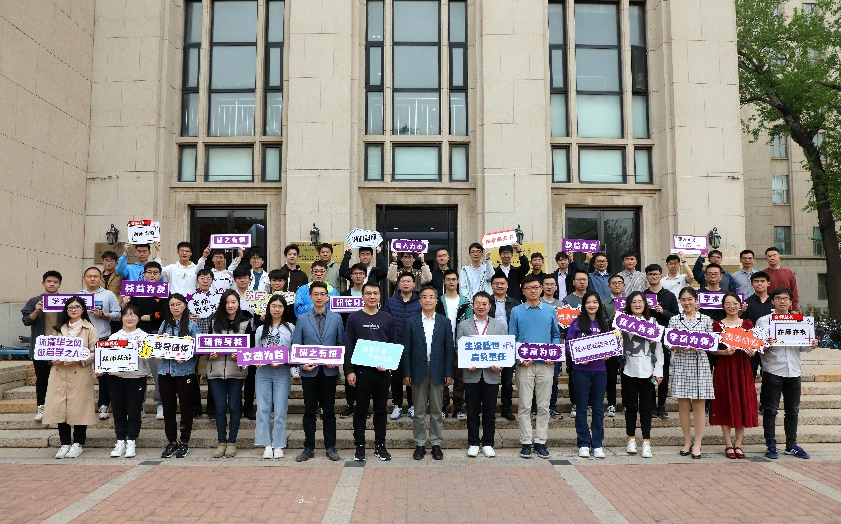
Team photo
Citations: Ning Zhang, Hongyang Jia, Qingchun Hou, Ziyang Zhang, Tian Xia, Xiao Cai, Jiaxin Wang, Data-Driven Security and Stability Rule in High Renewable Penetrated Power System Operation, in Proceedings of the IEEE, 2022, doi: 10.1109/JPROC.2022.3192719.
The paper introduction page on the Proceedings of the IEEE website: https://proceedingsoftheieee.ieee.org/data-driven-security-and-stability-rule-in-high-renewable-penetrated-power-system- operation/